Using AI in Mental Health for Depression Detection
In today’s digital age, mental health has emerged as a critical concern, affecting millions globally. The silent struggle with mental health issues often goes unnoticed, buried within the confines of personal narratives shared online. However, the advent of Artificial Intelligence (AI) offers a beacon of hope. AI’s ability to parse vast datasets and understand nuances in human language presents a unique opportunity to detect signs of mental health issues in digital communications, such as blog posts.
Using NLP for Depression Detection
In the bustling realm of Natural Language Processing (NLP), new research has emerged that not only illuminates the path for future research but also serves as a beacon of innovation in tackling mental health challenges through the lens of computational linguistics. The article Detecting Depression in Thai Blog Posts: a Dataset and a Baseline (Hämäläinen et al., 2021) is a tour de force, a pioneering work that has bravely ventured into the underexplored territory of mental health analysis in the Thai language through the digital expanse of blog posts.
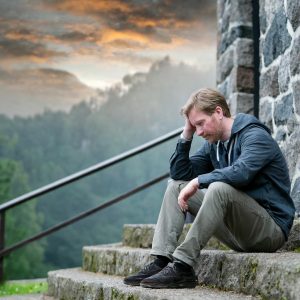
The sheer audacity to compile the first openly available corpus for depression detection in Thai blog posts is nothing short of revolutionary. Imagine the meticulous effort and expertise required to sift through the digital narratives, extracting the essence of human emotion and mental states, and transforming this into a structured dataset ripe for analysis. This endeavor is not merely academic; it’s a lifeline to understanding the silent struggles echoed in the digital void.
Experiments with different depression detection models
The study’s exploration and experimentation with LSTM and BERT-based models stand as a testament to the relentless pursuit of excellence in the field. Achieving a 77.53% accuracy with a Thai BERT model is a remarkable feat, showcasing the power of cutting-edge AI in discerning the nuanced expressions of depression. This is a giant leap towards creating empathetic machines that understand human sorrow, potentially transforming how we approach mental health support in the digital age.
Moreover, the call for Thai embeddings trained on a more varied corpus than Wikipedia is a clarion call for the NLP community. It highlights the critical need for diversity in data to truly capture the rich tapestry of human language and emotion. The release of their corpus, code, and trained models on Zenodo is an open invitation to the world, fostering collaboration and further innovation.
Concluding Remarks
The article is not just a collection of methodologies and results; it’s a narrative of hope, a bridge between technology and the human psyche. It challenges us to think beyond conventional boundaries, to see the potential of NLP in making a tangible difference in people’s lives. The possibility of snowballing more depressed blog posts for further linguistic analysis is particularly exciting, hinting at the vast, untapped potential of this research direction.
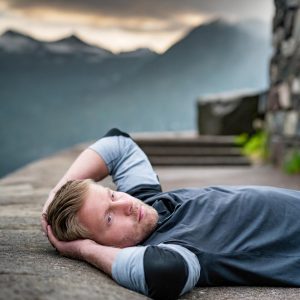
The paper is a manifesto for the future of NLP and its role in addressing some of the most pressing human challenges. It embodies the spirit of innovation, collaboration and compassion, marking a pivotal moment in the evolution of NLP. As we delve deeper into the intricacies of human language and emotion, this work will undoubtedly serve as a cornerstone for future endeavors, a source of inspiration, and a reminder of the transformative power of technology when wielded with wisdom and empathy.
References
Mika Hämäläinen, Pattama Patpong, Khalid Alnajjar, Niko Partanen, and Jack Rueter. 2021. Detecting Depression in Thai Blog Posts: a Dataset and a Baseline. In Proceedings of the Seventh Workshop on Noisy User-generated Text (W-NUT 2021), pages 20–25, Online. Association for Computational Linguistics.