Vernacular Voices: Leveraging NLP for Dialectal Text Analysis
In the rich tapestry of human language, dialects represent the vibrant variations that give voice to our diverse cultures and communities. However, these variations often present unique challenges in the realm of Natural Language Processing (NLP), where standard language has traditionally been the norm. In this blog post, we will uncover the cutting-edge techniques of dialect normalization, generation and detection. This post is all about celebrating the diversity of dialects in human communication!
Tackling Dialectal Text with Normalization
Imagine a world where the colorful diversity of dialects meets the precision of technology, where the rich tapestry of spoken heritage is woven seamlessly into the fabric of modern communication. This is not a mere fantasy, but a reality unfolding in the realm of Natural Language Processing (NLP), particularly in the picturesque landscapes of Finland.
Finland, a nation celebrated for its linguistic diversity, presents a unique challenge and an opportunity for NLP enthusiasts. The Finnish language, with its 23 distinct dialect varieties, serves as a vibrant playground for researchers aiming to bridge the gap between dialectal Finnish and its normative standard counterpart. The journey of normalization, transforming dialectal expressions into standard Finnish, is akin to alchemy in linguistics, turning the lead of regional variations into the gold of standardized communication.
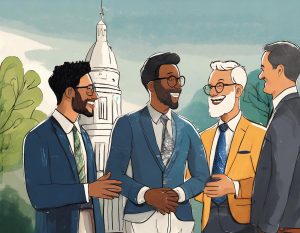
At the heart of this adventure are LSTMs and transformer models by Partanen et al. (2019), our knights in shining armor. These models, trained on a rich corpus of dialectal text, embark on a quest to lower the initial word error rate of the corpus from a daunting 52.89 to a mere 5.73. This leap is not just a number; it’s a testament to the power of machine learning in understanding and preserving the essence of human speech.
The methodology is akin to a master craftsman shaping raw materials into exquisite artifacts. Character-level NMT models learn the nuances of dialectal Finnish, translating it into the normative spelling with an elegance that belies the complexity of the task. The experiments, conducted across different granularities from words to sentences, reveal the intricate dance of context, syntax, and semantics in the realm of language.
Making Computers Speak in a Dialect
In the realm of computational creativity, the exploration of language as a canvas for innovation offers fascinating insights into the intersection of technology and cultural identity. A groundbreaking study (Hämäläinen et al. 2020) led by researchers from the University of Helsinki delves into the intricacies of Finnish dialects, presenting an ambitious project that not only challenges the computational norms but also enriches our understanding of linguistic diversity’s role in creative expression.
The study introduces a novel approach to adapting standard Finnish text into its numerous dialectal forms using character-level Neural Machine Translation (NMT) models. This endeavor is not merely an academic exercise but a crucial step toward preserving the rich tapestry of Finnish dialects, many of which are endangered. By incorporating over 20 different dialects, the research illuminates the complexity and beauty of Finland’s linguistic landscape.
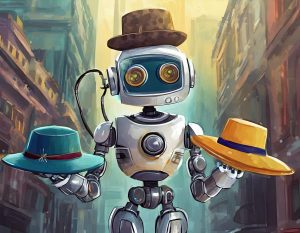
Central to this study is the examination of how dialect adaptation affects the perception of creativity in computer-generated poetry. The findings reveal a nuanced relationship between dialectical deviation from standard Finnish and creativity. Surprisingly, while poems in dialects far removed from the standard form were perceived as less creative according to conventional metrics, they were associated with higher creativity and originality on a word association test. This paradox underscores the subjective nature of creativity and the profound impact of linguistic diversity on its perception.
The implications of this research are manifold. For computational linguists and AI researchers, it offers a successful model for dialectal text adaptation that can be applied to other languages and contexts. For cultural historians and linguists, it provides a digital means of preserving linguistic heritage. And for the creatively inclined, it opens new avenues for exploring the interplay between dialect, identity, and artistic expression.
Identifying Spoken Dialects
The EMNLP published study, titled Finnish Dialect Identification: The Effect of Audio and Text (Hämäläinen et al., 2021), is a pioneering effort by researchers from the University of Helsinki. They have embarked on a fascinating journey to develop an automatic system capable of identifying Finnish dialects, leveraging both text and audio data.
Finnish, with its rich array of dialects, presents a unique challenge and an opportunity for linguistic research. The study’s significance lies in its comprehensive approach, combining audio and textual data to improve dialect identification accuracy significantly. The researchers trained their models on a dataset comprising 23 Finnish dialects, achieving a notable accuracy improvement when utilizing both text and audio information compared to text alone.
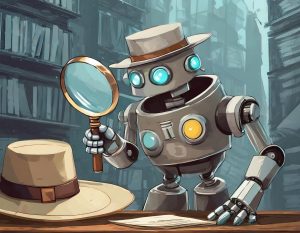
The implications of this research are profound, extending beyond academic interest to practical applications in enhancing automated speech recognition systems, facilitating dialect-specific content creation, and preserving linguistic diversity. By releasing their models and dataset openly, the team has not only contributed to the field of computational linguistics but also set a precedent for future collaborative research efforts.
This innovative study opens new avenues for exploring linguistic diversity using technology, offering insights into the complex interplay between spoken and written language forms. It underscores the importance of interdisciplinary collaboration in pushing the boundaries of what’s possible in language technology, promising exciting developments for Finnish and other languages with rich dialectal variations.
References
Niko Partanen, Mika Hämäläinen, and Khalid Alnajjar. 2019. Dialect Text Normalization to Normative Standard Finnish. In Proceedings of the 5th Workshop on Noisy User-generated Text (W-NUT 2019), pages 141–146, Hong Kong, China. Association for Computational Linguistics.
Hämäläinen, M., Partanen, N., Alnajjar, K., Rueter, J., & Poibeau, T. (2020). Automatic Dialect Adaptation in Finnish and its Effect on Perceived Creativity. In Proceedings of the 11th International Conference on Computational Creativity (ICCC’20). Association for Computational Creativity.
Mika Hämäläinen, Khalid Alnajjar, Niko Partanen, and Jack Rueter. 2021. Finnish Dialect Identification: The Effect of Audio and Text. In Proceedings of the 2021 Conference on Empirical Methods in Natural Language Processing, pages 8777–8783, Online and Punta Cana, Dominican Republic. Association for Computational Linguistics.