How to Conduct Human Evaluation in the Field of NLP?
I discuss two papers on human evaluation of NLP methods. The first presents a large problem in the field, whereas the latter studies how human evaluation is conducted and gives some suggestions on how it should be done in the future.
The Great Misalignment Problem in Human Evaluation
The Great Misalignment Problem, as discussed in the paper The Great Misalignment Problem in Human Evaluation of NLP Methods (Hämäläinen & Alnajjar, 2021a). underscores a critical issue in natural language processing (NLP) research: the misalignment among problem definition, proposed methods and human evaluation practices. This problem indicates a significant disconnection where the methods proposed do not align with the initial problem definition, and consequently, the human evaluation does not align with either the problem definition or the methods.
A survey of 10 papers from the ACL 2020 conference revealed that only one paper fully aligned in terms of problem definition, method and evaluation, highlighting the pervasive nature of this issue. This misalignment raises concerns about the validity and reproducibility of research findings in the field, suggesting that the current practices in human evaluation of NLP methods are not rigorous or reliable enough to accurately assess the advancements in the field.
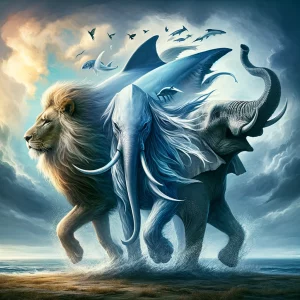
The Great Misalignment Problem refers to the inconsistency across three key components in NLP research: the problem definition, the proposed method, and the human evaluation. This means there’s often a disconnect where the methods used don’t directly address the defined problem, and the human evaluation criteria may not accurately measure the effectiveness of the proposed solution.
For example, a study might aim to improve the quality of a poem generation system but employs an evaluation method that focuses on rhymes, failing to align with the initial problem definition of enhancing overall quality. Not to mention that “improving quality of poems” is a very abstract definition and not concrete enough to be addressed in a scientifically rigorous fashion. This discrepancy can lead to misleading conclusions about the effectiveness of NLP techniques.
How is Human Evaluation Conducted?
In the realm of Natural Language Processing (NLP) and Computational Creativity (CC), human evaluation plays a pivotal role in assessing the success and impact of generated content, whether it be text, poetry, stories, or any form of creative output. A recent survey of human evaluation methods (Hämäläinen & Alnajjar, 2021b) across studies in these fields highlights the diversity and complexity of assessing creative natural language generation systems.
Human evaluations often employ scaled surveys, such as 5-point Likert scales, to measure various aspects of generated content, including its novelty, relevance, emotional impact, and syntactic correctness. However, the survey reveals a lack of consensus on best practices for conducting these evaluations, with many studies not clearly justifying their choice of evaluated parameters or the design of their evaluation methods.
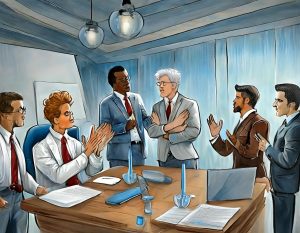
The paper advocates for a more rigorous and structured approach to human evaluation in creative NLG, emphasizing the need for clearly defined goals, concrete questions, multiple evaluation setups, and a thorough analysis of results. It also highlights the importance of reporting the evaluation process and potential biases transparently to enhance the validity and reproducibility of findings.
By adopting more standardized and thoughtful evaluation practices, researchers in NLP and CC can better understand the strengths and weaknesses of their systems, paving the way for more meaningful advancements in the field of creative language generation.
How Should Human Evaluation Be Done?
The paper by Hämäläinen & Alnajjar (2021b), stemming from a survey of creative NLG systems presented at INLG 2020 and ICCC 2020, emphasizes the importance of refining evaluation methodologies to better understand and assess the capabilities of such systems.
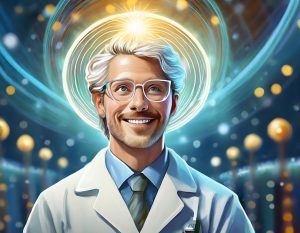
The authors recommend several critical strategies for future evaluations:
- Define Goals Clearly: The objectives of the generative system should be explicitly outlined to guide the evaluation process effectively.
- Concrete Questions: Evaluations should employ specific, concrete questions to minimize subjective interpretations of the system’s output.
- Test Evaluation Setup: Preliminary testing of the evaluation framework can uncover potential flaws or biases in the methodology, ensuring more reliable results.
- Utilize Multiple Evaluation Methods: Incorporating a variety of evaluation techniques can provide a more comprehensive assessment of the system’s performance.
- Transparent Reporting: The entire evaluation process, including potential biases, should be reported in detail to ensure the replicability and integrity of the findings.
- In-depth Analysis: Beyond surface-level statistics, a deeper analysis of the results can offer valuable insights into the system’s strengths and weaknesses, guiding future development.
These recommendations aim to enhance the scientific rigor and effectiveness of human evaluations in creative NLG, fostering a more nuanced understanding of these complex systems.
References
Mika Hämäläinen and Khalid Alnajjar. 2021a. The Great Misalignment Problem in Human Evaluation of NLP Methods. In Proceedings of the Workshop on Human Evaluation of NLP Systems (HumEval), pages 69–74, Online. Association for Computational Linguistics.
Mika Hämäläinen and Khalid Alnajjar. 2021b. Human Evaluation of Creative NLG Systems: An Interdisciplinary Survey on Recent Papers. In Proceedings of the 1st Workshop on Natural Language Generation, Evaluation, and Metrics (GEM 2021), pages 84–95, Online. Association for Computational Linguistics.